

With a non-significant result, we cannot reject that Null-Hypothesis. Using this test, we thus test the Null-Hypothesis that the respecting parameters are equal across the groups. The underlying logic is that if a model with the constraints imposed does not fit the data significantly worse than the previous model, the constraints do not make the model a significantly worse simplification of reality than the previous one. In the second approach, we don't check whether the model fits well, but whether it fits the data 'as well' as the previous model, by means of a chi-square difference test. If a certain model fits, it can be concluded that there is that particular type of invariance. Then based on the output of the model fit table the fit of the respecting models can be evaluated (here, the additional fit statistics, that you can chose under 'Additional Output' are important too). You can just fit the sequence of models by choosing the respecting model under 'Invariance testing' in the 'Multigroup CFA' tab. This can easily be done using jasp's CFA function. one where the factor loadings are constraint to be equal across groups) shows good fit, we can conclude that there is this form of measurement invariance (e.g. Some say that as soon as the model in question (e.g. There are different view points on when we can conclude that there is (a certain form of) measurement invariance. You will most likely not need this to be true and usually it is not tested for strict invariance in practice. This means that the groups share the same random measurement error. In a strict invariance model, the residual variances of the items are equal across groups too. This in turn allows to also meaningfully compare the means of the latent variables between groups. the 'base-level' scores on the items) are equal across groups. Scalar (or "strong") invariance means that also the intercepts (the score on an item when the score on the latent variable is 0, i.e. This, in turn, allows to formally (by means of a statistical test) compare structural parameters, such as regression coefficients or correlations between countries. This means that the latent variables share the same 'metric' or scale across groups. Next, in the metric (or "weak") invariant model the factor loadings are constraint to be equal across groups.

Both measures the same latent variable, but a comparison would be meaningless, without transforming one of the two scores first. To illustrate, think of comparing 2 groups on their average body temperature, where one group has been measured in Celcius and one in Kelvin.
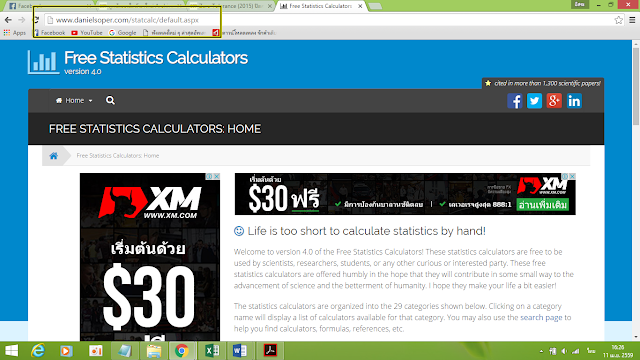
This means that the same latent variables are measured in the countries, but the way in which they are measured may still differ, such that meaningful comparisons between groups are impossible. factor loadings, intercepts, and residual variances) are allowed to vary between groups, although the factor structure of the model is identical. First, in the configural model all parameters (i.e. In order to test for measurement invariance, you need to fit a sequence of models. This is indeed a good question, and this will be a relatively long answer accordingly.
